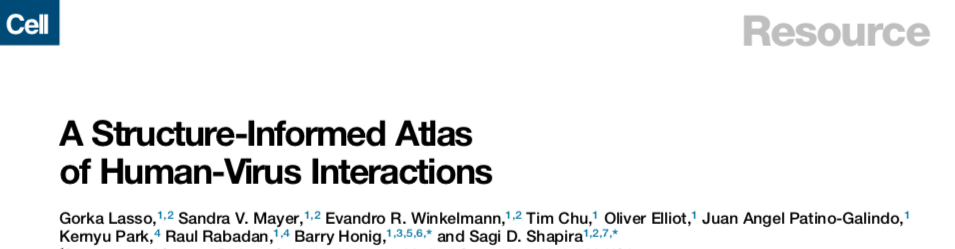
Viruses are intracellular obligate pathogens that rely on the molecular machinery of the host to fulfill their life cycle. In order to achieve this, viruses interact with host proteins, rewiring the molecular network of the host for their own benefit. Therefore, understanding how viruses interact with the host protein network is of particular interest to understand the molecular principles behind viral infection.
Despite the relevance of these interactions, our knowledge is exceedingly sparse, and very few viruses have been studied extensively experimentally. Partly, due to the difficulty to study such interactions in an experimental setting.
With this in mind, I have tuned PrePPI, a well-known algorithm developed at Barry Honig’s lab, to predict protein protein interactions (PPIs). PHIPSTER (Pathogen Host Interactome Prediction using STructurE similaRity) evaluates potential direct PPIs between a viral and a host protein by considering domain-domain contacts and peptide-domain contacts.
We applied our predictive algorithm on a viral dataset comprising 1,001 human-infecting viruses (12,237 viral proteins) against the human proteome (~20,000 human proteins), generating ~282,000 high confidence viral-host PPI predictions.
Overall, our high confidence predictions have a good predictive performance and overlap with high-throughput experimental methods. Our approach can have multiple applications, such as understanding the role of each viral protein, revealing the host biological pathways underlying human infection, and discovering functional relationships between viruses.
Our predicted Zika virus (ZIKV) protein interactome involves human proteins whose biological role are very much related to the phenotype of the Zika virus infection observed in the latest outbreak in the Americas. Moreover, we identified a particular druggable human protein acting as rheostat of viral infection that might lead to a new strategy to treat zika virus infection.
We also identified particular viral-host PPIs that are able to classify the known the oncogenic potential of human papilloma viruses (HPVs). These viruses are the leading cause of cervical cancer in women, but not all HPVs have the same ability to induce cancer. Our predictions discern between high risk HPVs and low risk HPVs, enabling the classification of other HPVs whose oncogenic potential is not known yet and hopefully bringing some additional light (since HPVs have already been widely studied) into the molecular processing underpinning cervical cancer.
Having now all viruses described by their (predicted) set of viral-host PPIs and host biological functions important during viral infection, there is some cool stuff we can do such as identifying functional relationships between viruses independently of their evolutionary origin. Throughout evolution viruses diversify, by-passing the host’s antiviral mechanisms, and explore new infection routes that can give them an adaptive advantage over the host (the so-called arms-race). Following this, it is possible that unrelated viruses have converged into certain routes, which could be an interesting target in drug therapy to treat multiple infectious diseases at once.
It is important to remark that this work reports predictions and further experimental work should follow up to explore the hypotheses generated here. Theoretical and experimental work beautifully together when theory generates hypotheses that are tested experimentally and these results are used to further refine the theoretical model in an feedback loop.
Considering that “All models are wrong, but some are useful” (George Box), I would like to believe that some of our predictions are correct and contribute to understand viral pathogenesis.